Navigating Token Limits
By now, you know LLMs are the AI powerhouses trained on heaps of data, and prompts are what enable you to make the most out of them.
However, it’s important to learn that different LLMs have specific token limits that define their performance. Ideally, when you’re creating your prompt, you need to ensure that you’re not crossing these token limits. Let’s understand this concept quickly.
- Token Limits: These dictate how many tokens an LLM can handle in one go.
- Estimated Word Counts: This refers to the approximate number of words that can fit within a model’s token limit. It helps you gauge how much content you can generate or process.
If you try copy-pasting a long Wikipedia article, you might notice an error.

Think of token and word counts as your LLM's capacity. While tokens define the technical limit, estimated word counts translate this into a more human-understandable measure.
Why It Matters: Knowing the estimated word count helps you manage your input prompts and outputs more efficiently.
Comparative Analysis: Token and Estimated Word Counts in a Few Leading LLMs
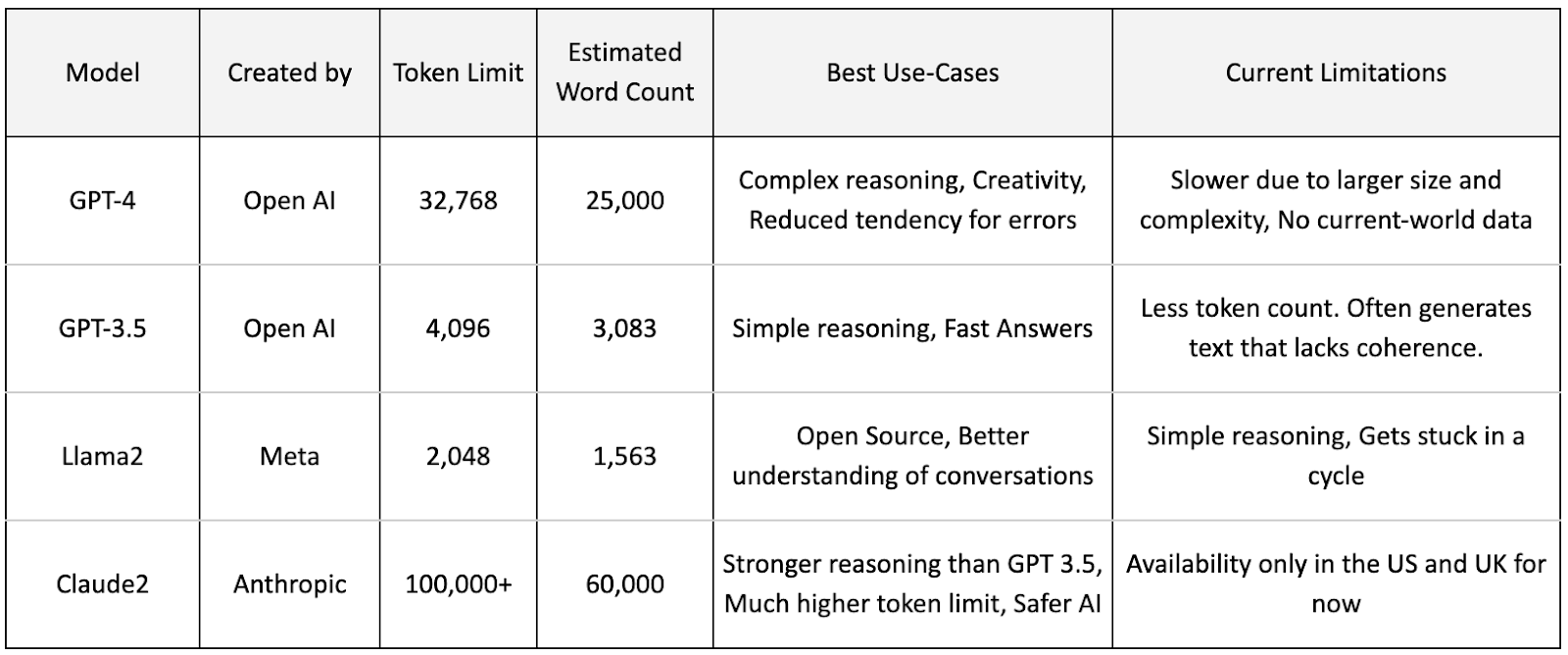
LLMs process prompts based on vast data sets, leading to token limits that cap the amount of text (input and output) they can handle in one interaction. Understanding these limits is essential for crafting effective prompts without exceeding the model's processing capacity.
- A nuanced understanding of token limits is key to maximizing your LLM interactions.
- Tailored document management strategies can help navigate the constraints imposed by token limits.
- Employing these techniques enhances the effectiveness and accuracy of information processing, ensuring a more satisfactory experience with LLM technologies.
Navigating Token Limit Constraints with Prompt Engineering
While challenges such as privacy and extensive manual effort still exist, the problem statement of navigating token limits through efficient prompting can be broken down into a few components.
- Understanding Token Limits:
- Simple Queries: Asking an LLM, "How many tokens can you support?" can quickly reveal its capacity.
- Competitive Edge: LLM services often highlight their token support capabilities as a competitive advantage. For instance, ChatGPT-4’s token range is from 8,192 to 128,000, showcasing significant variability and adaptability. Newer LLMs boast a much higher token limit (check Claude 3 by Anthropic for instance).
- Strategies for Managing Excess Length: When dealing with documents that exceed these limits, a few strategic approaches can help maintain accuracy and reduce the risk of losing essential information:
- Truncate or Divide: Break down the document into sequential sections, ensuring each is within the token limit.
- Eliminate Irrelevancies: Remove information not relevant to your main focus to streamline the content.
- Retrieval-Augmented Generation: Utilize techniques that allow the LLM to access external information, effectively extending the document size it can handle. Our next module is going to be pretty much about this.
Additional Links on Token Limits
While the foundational knowledge provided is adequate for course progression, further exploration of tokens is available in the documentation linked below.